Putting Machine Learning to Work to Speed Up Image Analysis
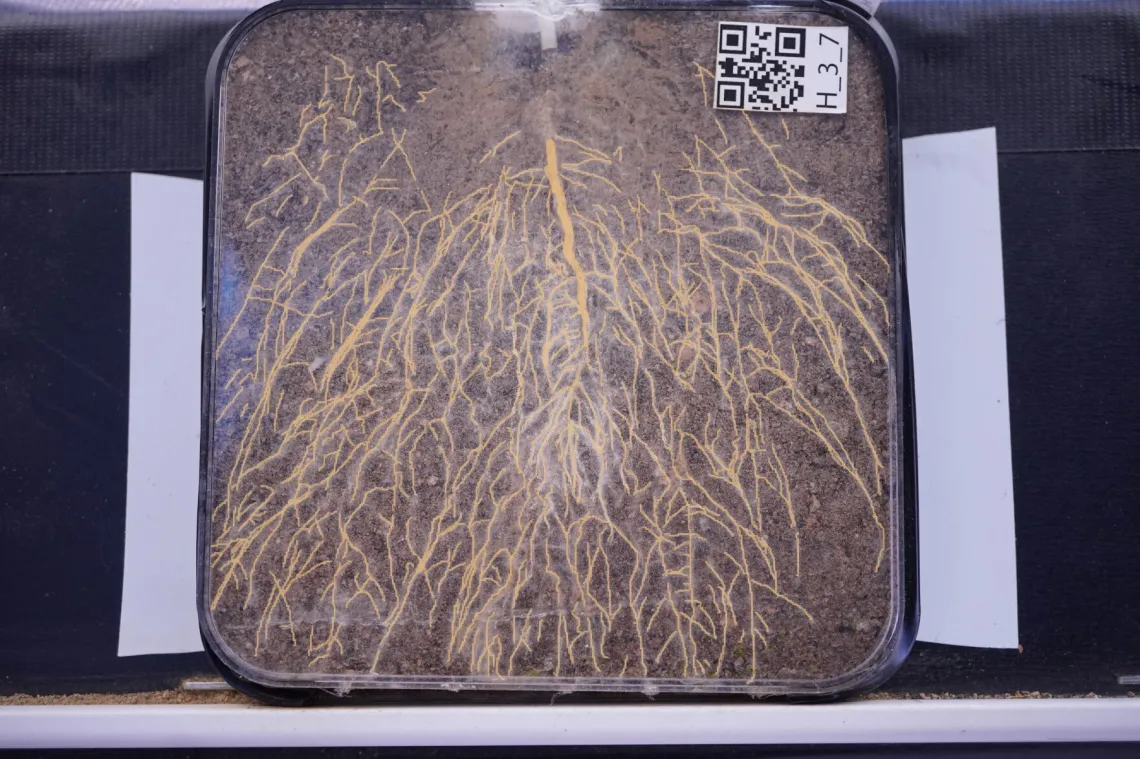
Data collection for a lab can be time consuming and tedious. Data, and a lot of it, is necessary however in our pursuit of a scientific conclusion. We are surrounded by technology that automates our daily tasks making our lives so much easier. With all of this technology, there has to be a more efficient way for us to collect and manage data. How can we make the data we collect do the work for us?
That is the question Kamila Murawska-Wlodarczyk asked herself when she began her lab in the Spring of 2021. Wlodarczyk is a PhD student working on behalf of the University of Arizona Superfund Research Center (UA SRC), who is also a current Roots for Resilience participant for the Spring 2022 cohort. She is working on Project 5 for the UA SRC, which is funded by the National Institute of Environmental Health Sciences (NIEHS) and will be studying the effects of hazardous waste on the environment as well as its impact on human health. Project 5, specifically, will study the potential use of plants to clean up mine tailings. By monitoring plant growth in different soil compositions, we can learn about how to effectively phytoremediate contaminated mine sites.
Wlodarczyk spends an inordinate amount of time measuring leaf growth for Project 5. The process is quite laborious and includes many tedious steps. She may spend up to several hours a week taking pictures of the plants and tracing new growth.
Wlodarcyzk is now working with the Data Management and Analysis Core (DMAC) in order to better consolidate the analysis workflow and data. Michelle Yung, a software engineer at the Data Science Institute, works on behalf of DMAC and assists Wlodarczyk on the data analysis portion of her research. Yung is currently building an app using Streamlit, a popular tool that is used for building web-based interactive applications. The Streamlit app will allow researchers with similar data to Wlodarczyk to be able to upload their files and run root and leaf detection analysis themselves without having to install any software. All leaf and root data can be easily uploaded to CyVerse and the app could be accessed and run from the CyVerse site as well.
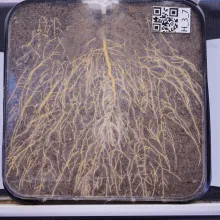
The process for machine learning begins with the traced rhizoboxes. Yung enlisted the help of Hayley Limes, an undergraduate student working in Raina Maier’s lab alongside Wlodarcyzk, to label the images of the rhizoboxes. She then highlights the leaf and root structure images using LabelStudio, an open source tool which allows for multiple users to collaboratively label data for machine learning. These annotated images are then exported to train, or build, the machine learning model that is capable of rapidly performing leaf and root segmentation on new images that it has never seen before. It also recognizes the QR code on the upper right-hand side of the rhizobox and renames the images from the default cryptic names created by the camera into something more descriptive about the unique identity of each plant. This makes it much easier for the team to organize and manage their data by cutting out the manual work of having to rename each file.
The goal of the machine learning model, and the Streamlit app, is for Wlodarczyk and her lab members to process their data, independently. When they have new images to analyze, they upload them to CyVerse, run the leaf segmentation model on the new images, as well as visualize the results. The more this application is utilized by Kamila and her team, the models will be further refined and optimized to improve the segmentation accuracy. Automating the detection of the leaves and root structures in this manner will make the analysis reproducible. Additionally the resultant model can be shared with community members that need to perform similar analysis, allowing them to readily process large amounts of data on other computational platforms such as the UArizona campus High Performance Computing (HPC) system.
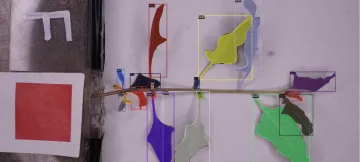
Yung says, “Automating the leaf and root detection will save researchers time in future experiments, and also would allow for future experiments to be scaled up in size because much of the manual work would be eliminated. Ideally this experiment could become something bigger if other researchers are doing similar studies.”
Thanks to the development of this Streamlit app by Yung, and further research conducted by the UA SRC, we are one step closer to remediating mine pollution and related health risks as well as bolstering the use of machine learning in this field of research.
Michelle will be hosting a webinar on data and apps to build custom web applications. The webinar will take place on August 26th at 10 a.m. MST. For more information or to register, visit https://cyverse.org/webinars.
For more information about the Data Science Institute, the Roots for Resilience (R4R) program, University of Arizona Superfund Research Center visit:
https://datascience.arizona.edu/
https://datascience.arizona.edu/r4r
https://superfund.arizona.edu/about/overview
The University of Arizona Superfund Research Center is supported by NIEHS award number #P42ES004940.
CyVerse is supported by the National Science Foundation under Award Numbers DBI-0735191, DBI-1265383, and DBI-1743442.